Software Testing
![PH_wp_[EN]_Blog listing - banner](https://qestit.com/hubfs/Website/Web%20pages%20photos/PH_wp_%5BEN%5D_Blog%20listing%20-%20banner.jpeg)
![PH_wp_[EN]_Blog listing - banner](https://qestit.com/hubfs/Website/Web%20pages%20photos/PH_wp_%5BEN%5D_Blog%20listing%20-%20banner.jpeg)
![PH_wp_[EN]_Blog listing - banner](https://qestit.com/hubfs/Website/Web%20pages%20photos/PH_wp_%5BEN%5D_Blog%20listing%20-%20banner.jpeg)
![PH_wp_[EN]_Blog listing - banner](https://qestit.com/hubfs/Website/Web%20pages%20photos/PH_wp_%5BEN%5D_Blog%20listing%20-%20banner.jpeg)
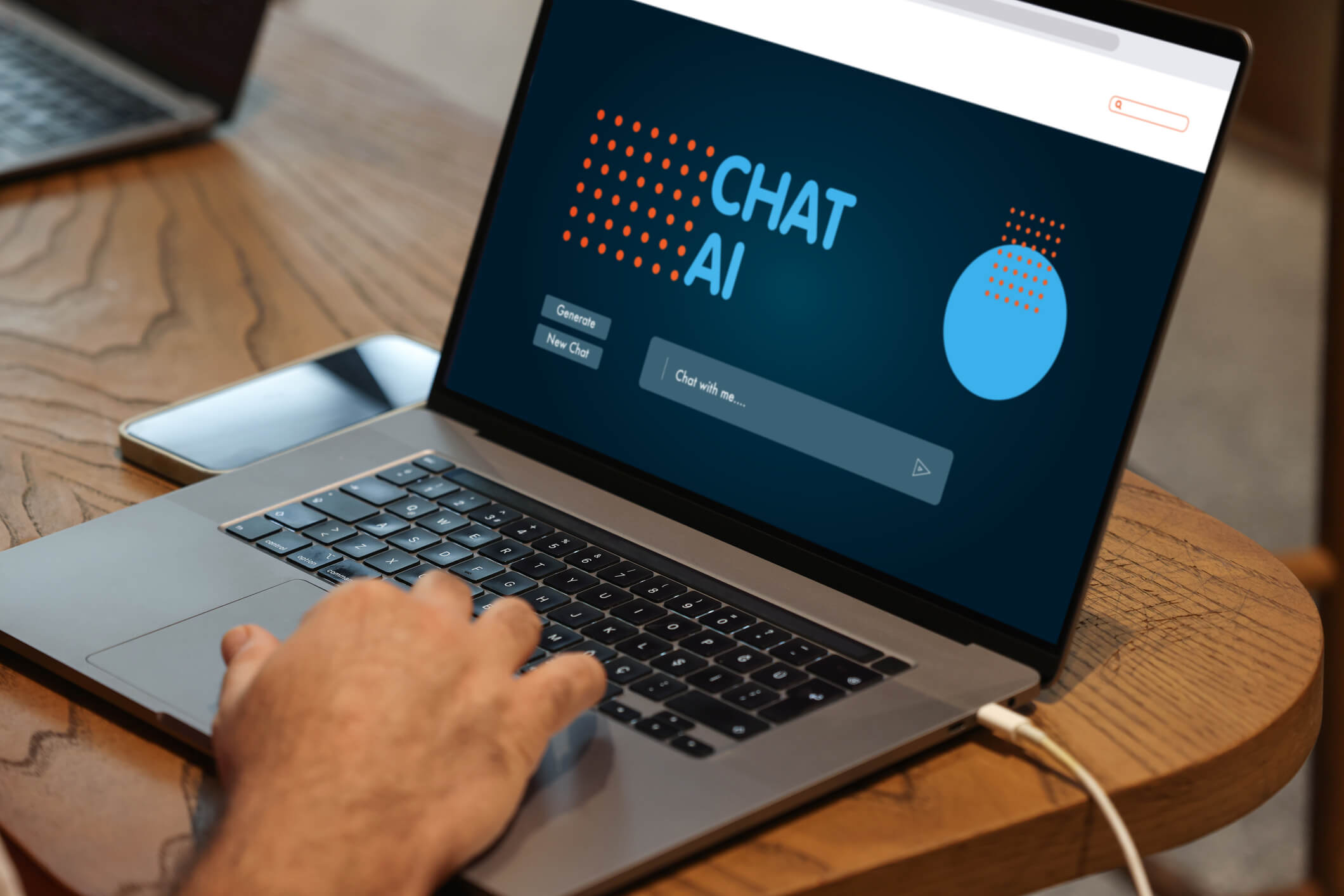
AI Testing
Empower your business with strategic AI integration for a competitive edge, smoother workflows and empowered teams.
Provide Your Information
AI helps foster a culture of innovation and agility
The transformative potential of AI is reshaping industries, enabling businesses to meet the demands for innovation, enhance customer satisfaction, and ensure the integrity of robust, secure systems. Tailored AI solutions provide the agility and precision necessary to maintain leadership in any field. By enhancing operational efficiency, insights, and aligning with strategic business goals, AI can significantly reduce costs and improve outcomes.
These AI initiatives represent more than technological advancements, they are a powerful force to drive businesses towards a future of smoother workflows, empowered teams, and leadership in innovation. Embedding AI seamlessly into operations fosters a culture of innovation and excellence, prioritizing the client's strategic objectives.
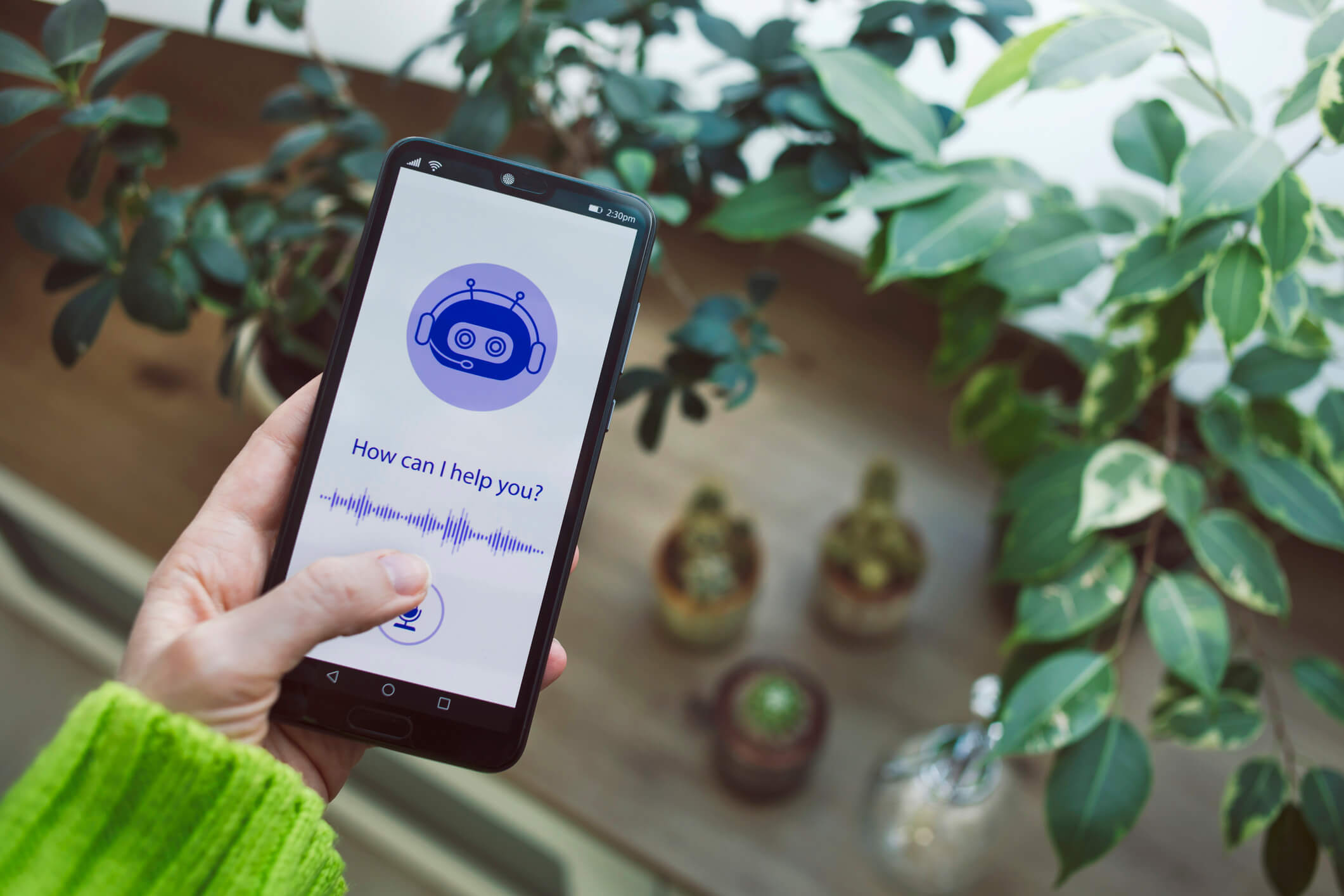
Dedicated quality assurance for AI systems is critical, ensuring superior performance and security.
- QA for Machine Learning Systems - Ensuring operational efficiency and safety across functions from image recognition to data analytics.
- QA for Generative AI Applications - Catering to a broad range of user needs with both open-source and proprietary AI models.
Our Tailored training programs with Generative AI Expertise are designed to bolster AI capabilities within teams, focusing on the practical application from the planning stage to deployment, with a strong emphazis on data security and the protection of proprietary information.
By adopting AI solutions that are specifically tailored to the needs and goals of the business, you can maintain a competitive edge and stay ahead in the rapidly evolving digital landscape.
Optimize your AI testing initiatives
Need help with AI?
Get in touch with our experts to understand the intricacies of AI tools and how your project can benefit from them.
Provide Your Information
Common questions about AI Testing
AI testing is the process of validating and verifying AI models and systems to ensure they function as intended and produce reliable, unbiased, and ethical outcomes. It's important because AI systems can be complex and behave unpredictably. Effective testing ensures that AI behaves as expected under a variety of conditions, complies with regulatory standards, and does not cause harm or act in ways that could damage a company’s reputation or finances.
AI testing is different from traditional software testing due to the inherent unpredictability and learning capabilities of AI systems. While traditional software has a deterministic nature with predefined inputs and outputs, AI systems learn from data, adapt over time, and may produce different outputs given the same input. This requires dynamic testing approaches that can accommodate such variability and continuous learning.
- Grasping Application Scope: Understanding the intended use, limitations, and context of the AI application is crucial for creating relevant test cases.
- Multi-Level Testing Strategies: This involves a tiered approach to testing, from unit tests to integration and system-level evaluations, to thoroughly examine the AI's functionality and security.
- Outcome-Focused Testing: Concentrating on the AI's outcomes and how they fulfill real-world requirements, rather than just the underlying algorithms.
- Risk Mitigation and Reliability: Identifying potential risks, including data biases and security vulnerabilities, and implementing strategies to address them to ensure the AI system's integrity.
- Continuous Testing Commitment: Regularly revisiting the AI system for testing, especially for those deployed in changing environments, to catch any deviations from expected performance early on.
Automation in AI testing can greatly enhance the efficiency and coverage of tests. It can be used to run repetitive and complex test cases, handle large datasets efficiently, and perform tests consistently. Automated tests can be quickly adapted to changes in AI models and can run 24/7, providing continuous feedback and validation.
- Developing robust testing frameworks that can adapt to the AI's learning nature.
- Using synthetic data generation to enhance test coverage.
- Applying explainable AI techniques to interpret the model's decision-making process.
- Establishing clear testing metrics and benchmarks for performance, fairness, and reliability.
- Engaging in cross-disciplinary collaborations to ensure ethical and regulatory considerations are met.
More useful resources about software testing
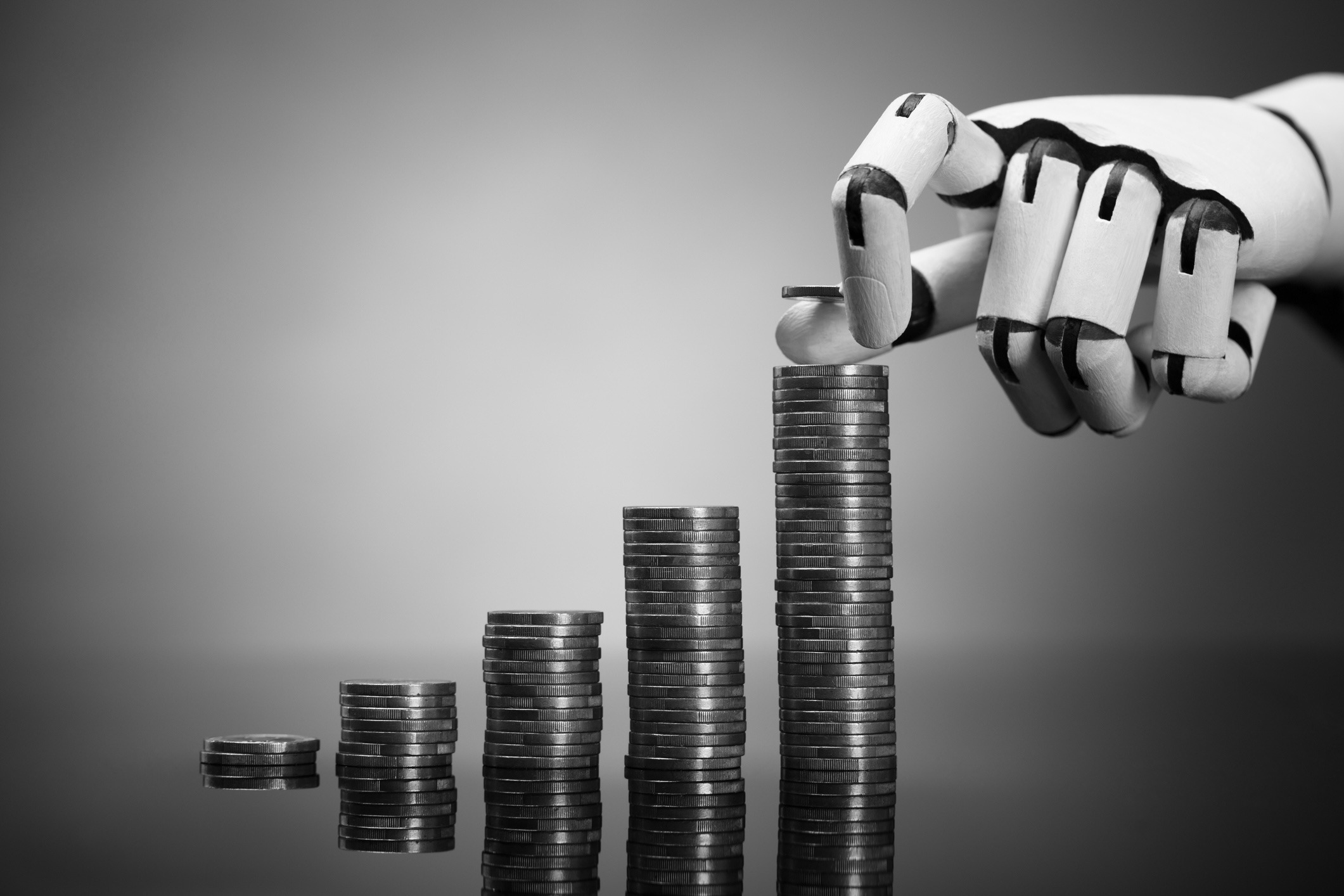
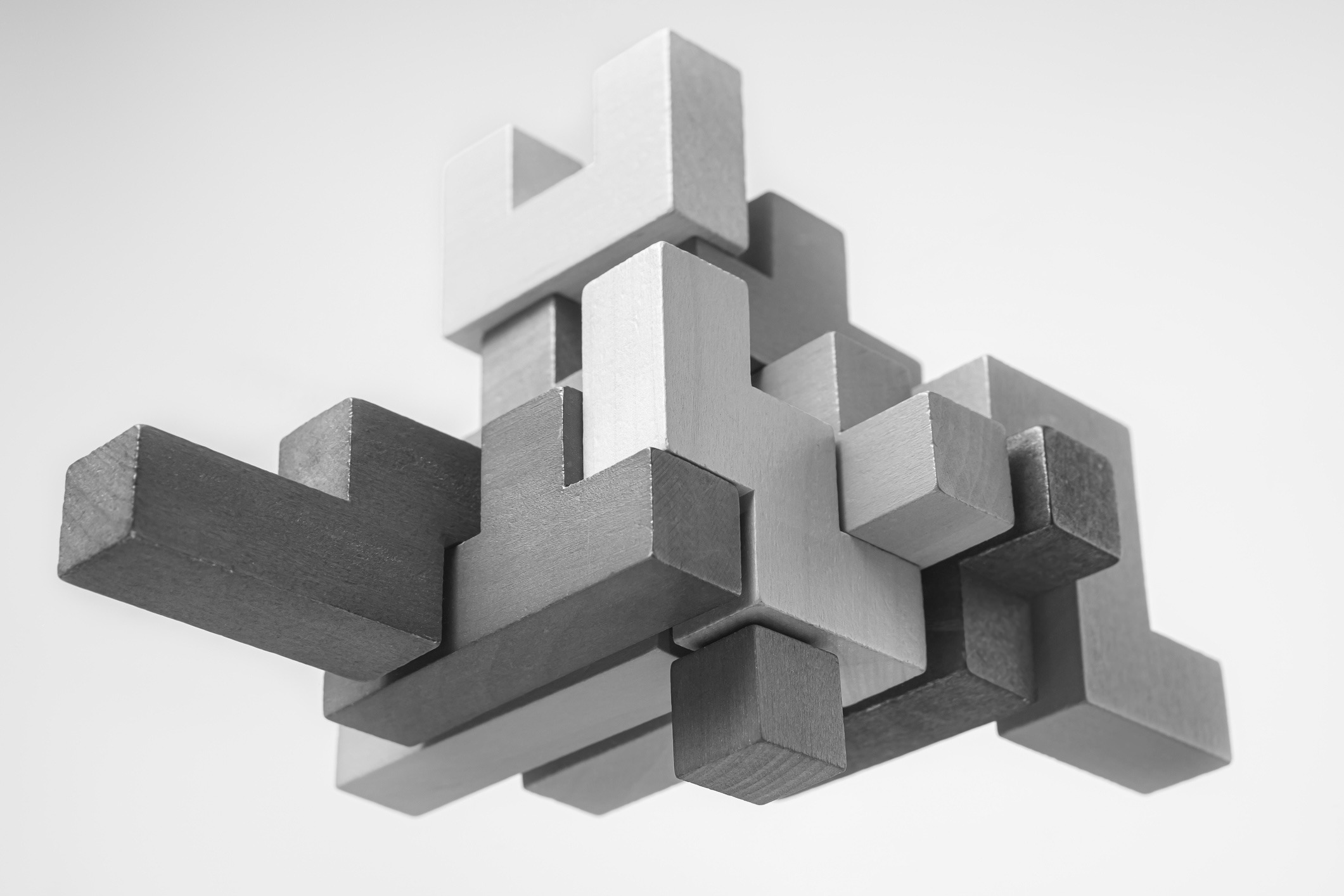
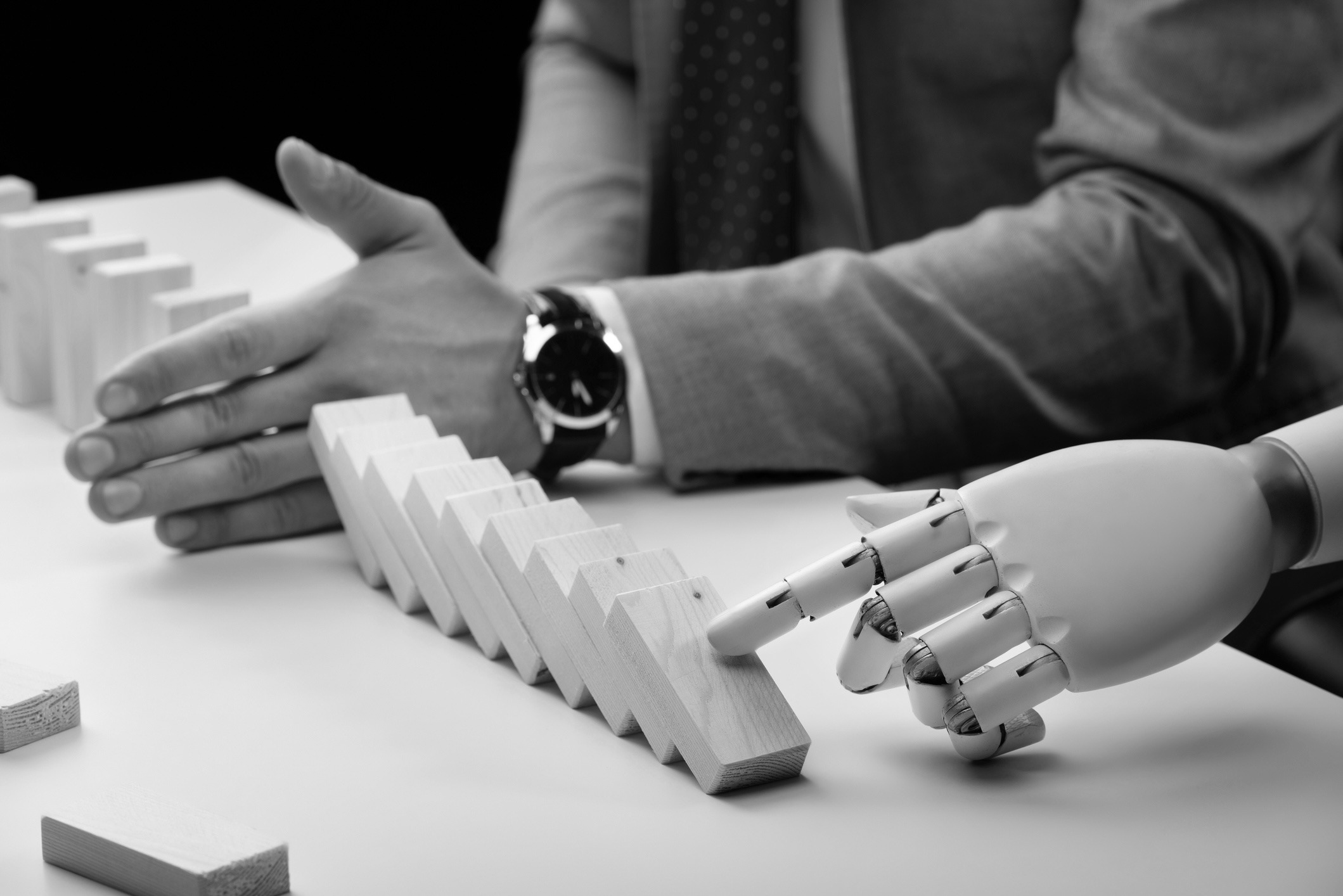