Software Testing
![PH_wp_[EN]_Blog listing - banner](https://qestit.com/hubfs/Website/Web%20pages%20photos/PH_wp_%5BEN%5D_Blog%20listing%20-%20banner.jpeg)
![PH_wp_[EN]_Blog listing - banner](https://qestit.com/hubfs/Website/Web%20pages%20photos/PH_wp_%5BEN%5D_Blog%20listing%20-%20banner.jpeg)
![PH_wp_[EN]_Blog listing - banner](https://qestit.com/hubfs/Website/Web%20pages%20photos/PH_wp_%5BEN%5D_Blog%20listing%20-%20banner.jpeg)
![PH_wp_[EN]_Blog listing - banner](https://qestit.com/hubfs/Website/Web%20pages%20photos/PH_wp_%5BEN%5D_Blog%20listing%20-%20banner.jpeg)
![PH_wp_[EN]_Blog listing - banner](https://qestit.com/hubfs/Website/Web%20pages%20photos/PH_wp_%5BEN%5D_Blog%20listing%20-%20banner.jpeg)
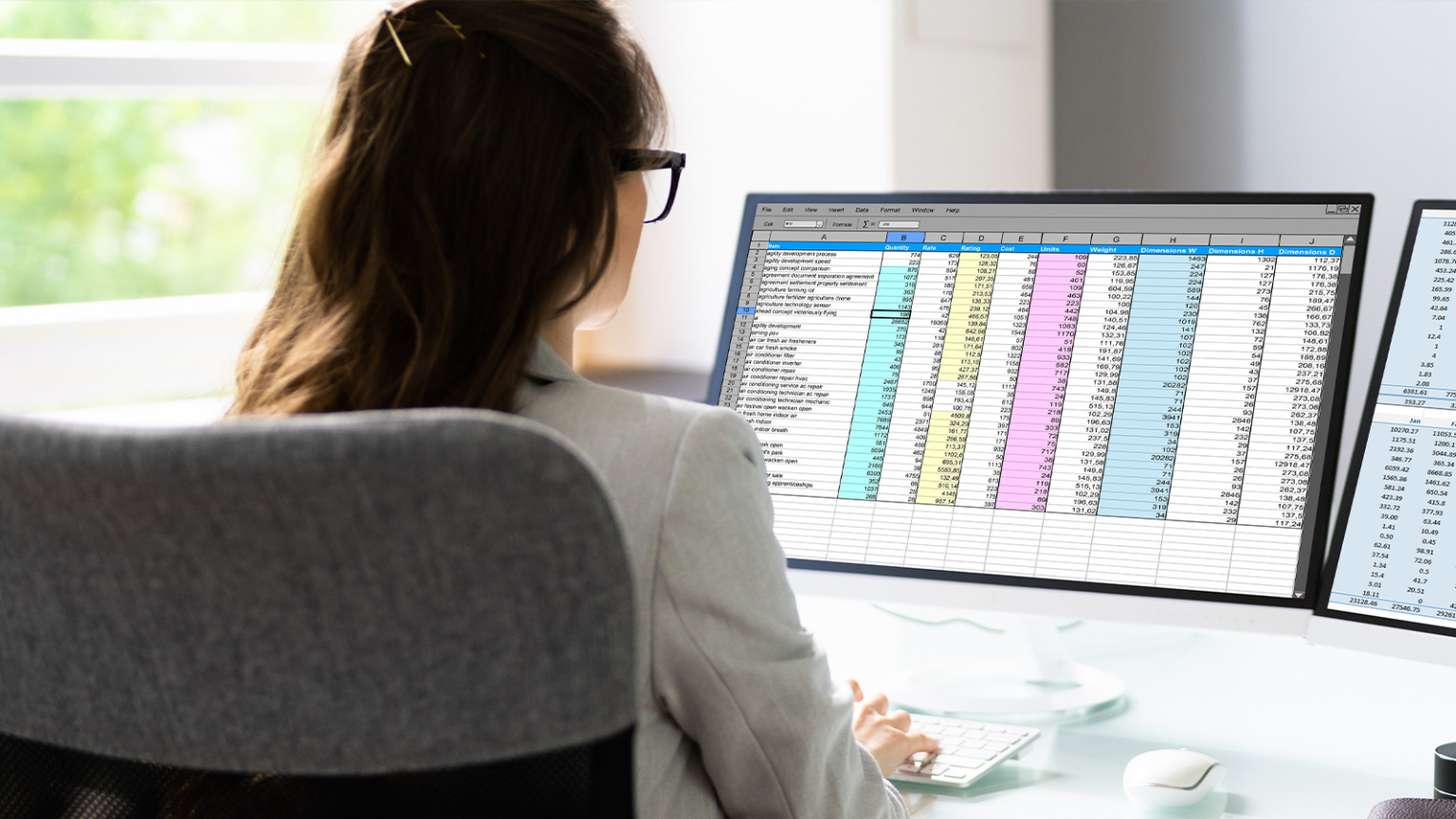
Data Reconciliation
Fragmented or inconsistent data can make decision-making challenging. Data reconciliation consolidates information into a unified, accurate dataset, providing a reliable foundation for strategic and operational success.
Provide Your Information
Ensuring Accurate Data for Reliable Operations
Businesses often face challenges reconciling large volumes of data across inconsistent formats and complex integrations, as even minor errors can have major repercussions. Data reconciliation resolves these issues by unifying and verifying information, ensuring quality, compliance, and security.
Our framework addresses these challenges with advanced parallel processing and streamlined data mapping solutions. By leveraging reusable scripts and mapping sheets, we simplify transformations, identify discrepancies, and ensure smooth data migrations while upholding data integrity and supporting critical business operations.
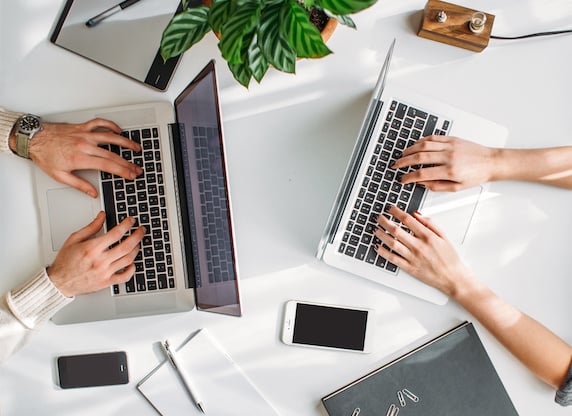
Reusable scripts standardize data reconciliation, enhancing security, consistency, and efficiency while minimizing errors during pre-production data transfers. Mapping sheets provide a clear framework for complex transformations, ensuring precision and facilitating collaboration among stakeholders.
With several major data reconciliation projects successfully completed, including the migration of portfolios from legacy systems to modern platforms, our expertise is proven. Each of these projects typically encompassed an average of 5,000 tables. For banking clients, we managed up to 7,000 tables, millions of customer and related products with tables containing up to 200 million records. Throughout, we maintained the highest standards of data quality and integrity. Additionally, we successfully executed numerous medium and small-scale projects.
Transform migrated data into a unified, error-free resource
Verification Maintaining data accuracy and consistency between source and target databases to preserve operational reliability and integrity and ensure seamless functionality.
Achieve seamless integration
Confidently navigate data migrations with solutions designed to unify, verify, and optimize your data. From enhancing accuracy to ensuring compliance, we help you achieve reliable results and maintain business continuity.
Provide Your Information
Common questions about data reconciliation
Data reconciliation is the process of ensuring that data between two systems, typically a source and a target, are consistent and accurate after migration or integration. It is very important as it ensures data integrity, accuracy, and consistency, which are crucial for maintaining business operations, making informed decisions, and ensuring compliance with regulations.
In data reconciliation, common types of discrepancies include:
- Missing Records: Data present in the source system is absent in the target system or vice versa.
- Extra Records: Data exists in the target system that is not present in the source system.
- Data Mismatches: Values in corresponding fields differ between the source and target systems.
- Duplicate Records: Unintended duplicate entries appear in one or both systems.
- Column Discrepancies: Differences in column structures, such as missing columns or mismatched data types.
- Aggregation Errors: Inaccurate totals or summaries when comparing aggregated data between systems.
These discrepancies can affect data integrity and operational performance, making early identification and resolution critical.
Parallel processing improves data reconciliation by significantly enhancing speed, efficiency, and scalability. It allows reconciliation tasks to be executed simultaneously across multiple processors or threads, enabling the system to handle large volumes of data in a shorter timeframe. This approach reduces bottlenecks and ensures that even complex or resource-intensive reconciliations are completed efficiently. By distributing workloads, parallel processing also minimizes downtime and supports real-time or near-real-time reconciliation for time-sensitive operations.
Mapping sheets define the relationships and transformations between source and target tables, serving as a blueprint for the reconciliation process. They guide the reconciliation tool to accurately map, transform, and compare data, ensuring consistency and alignment with business requirements. By detailing field mappings, validation rules, and special requirements, mapping sheets help streamline complex transformations and minimize errors during data migration or reconciliation.
More useful resources about QA
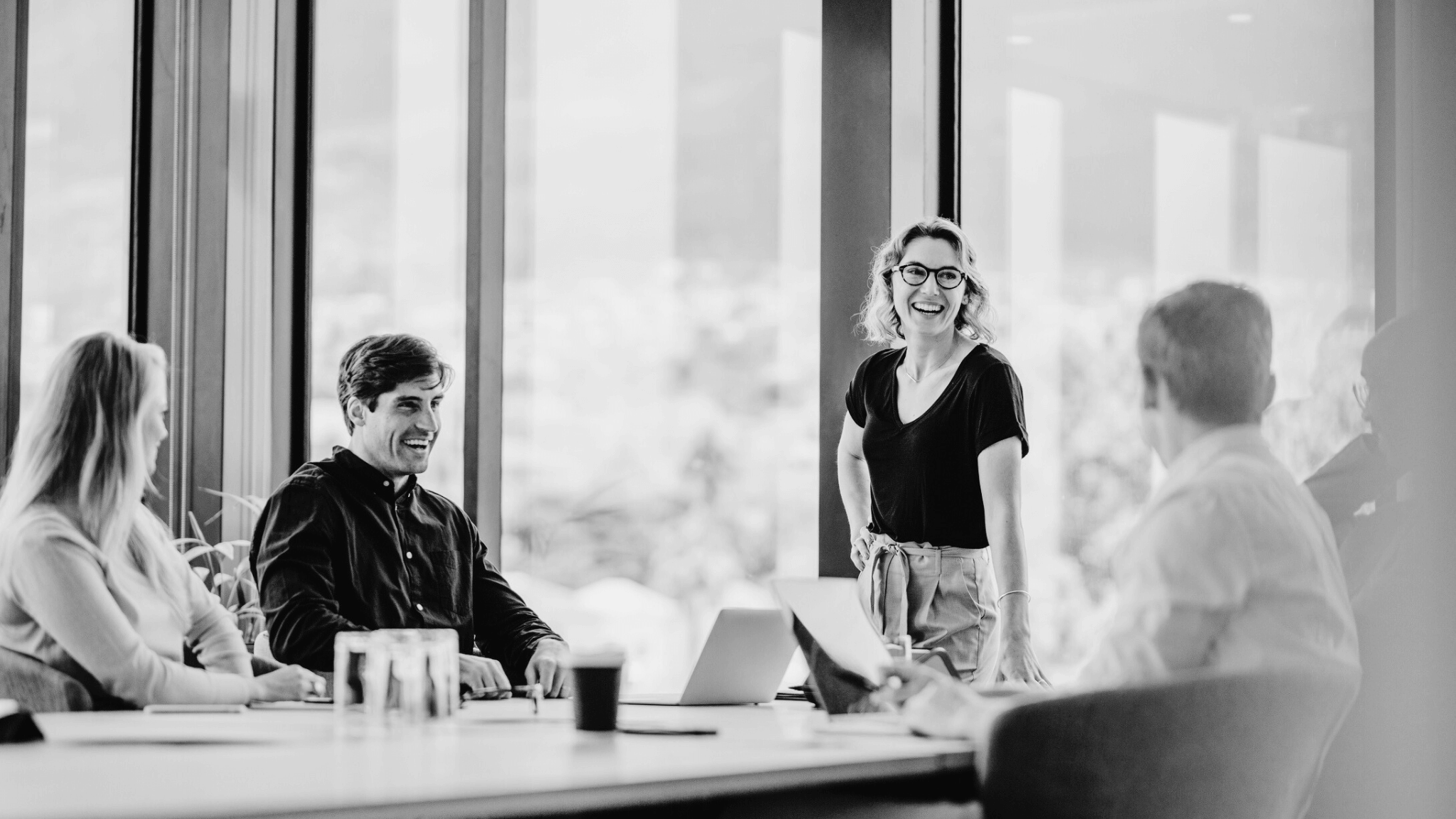
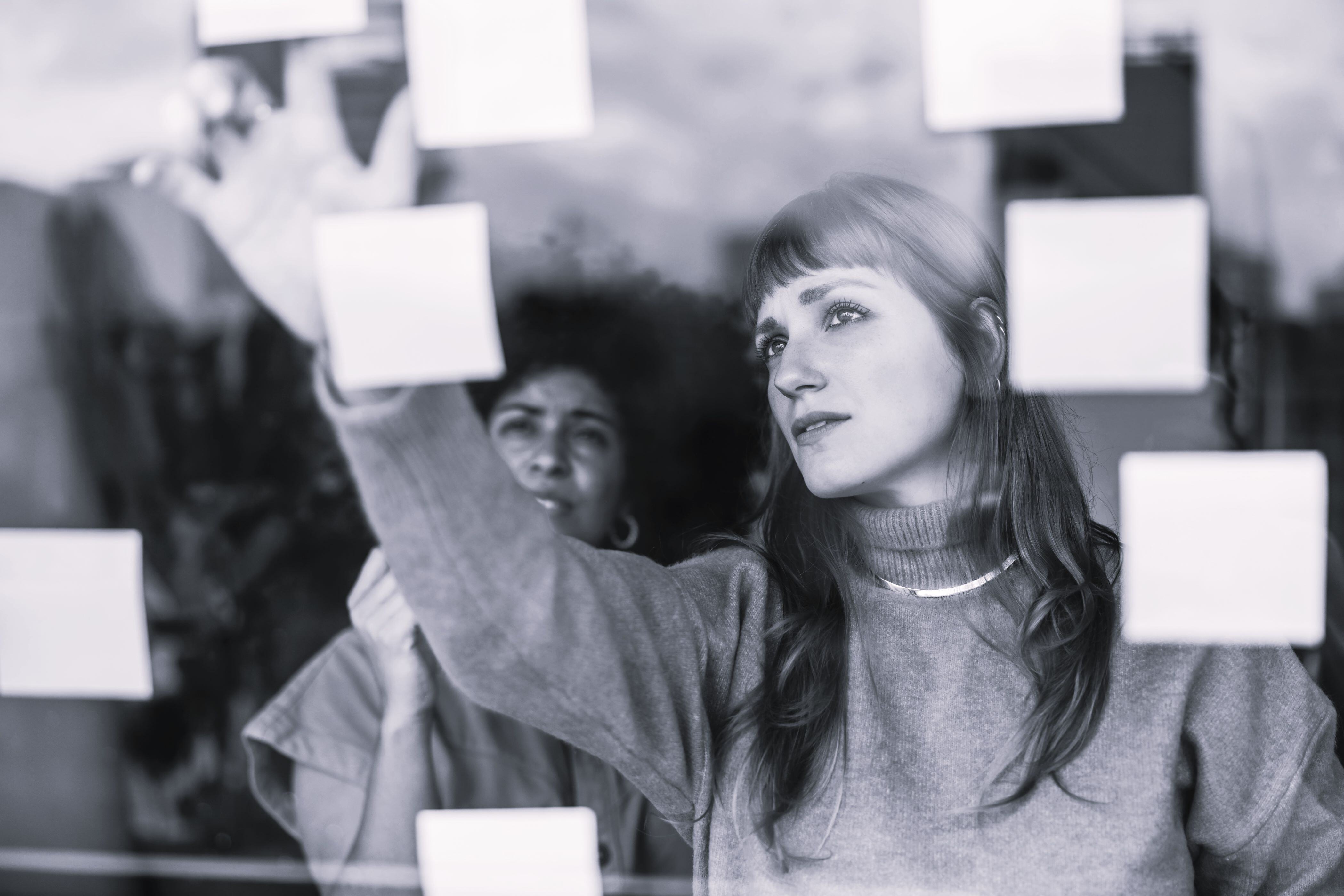
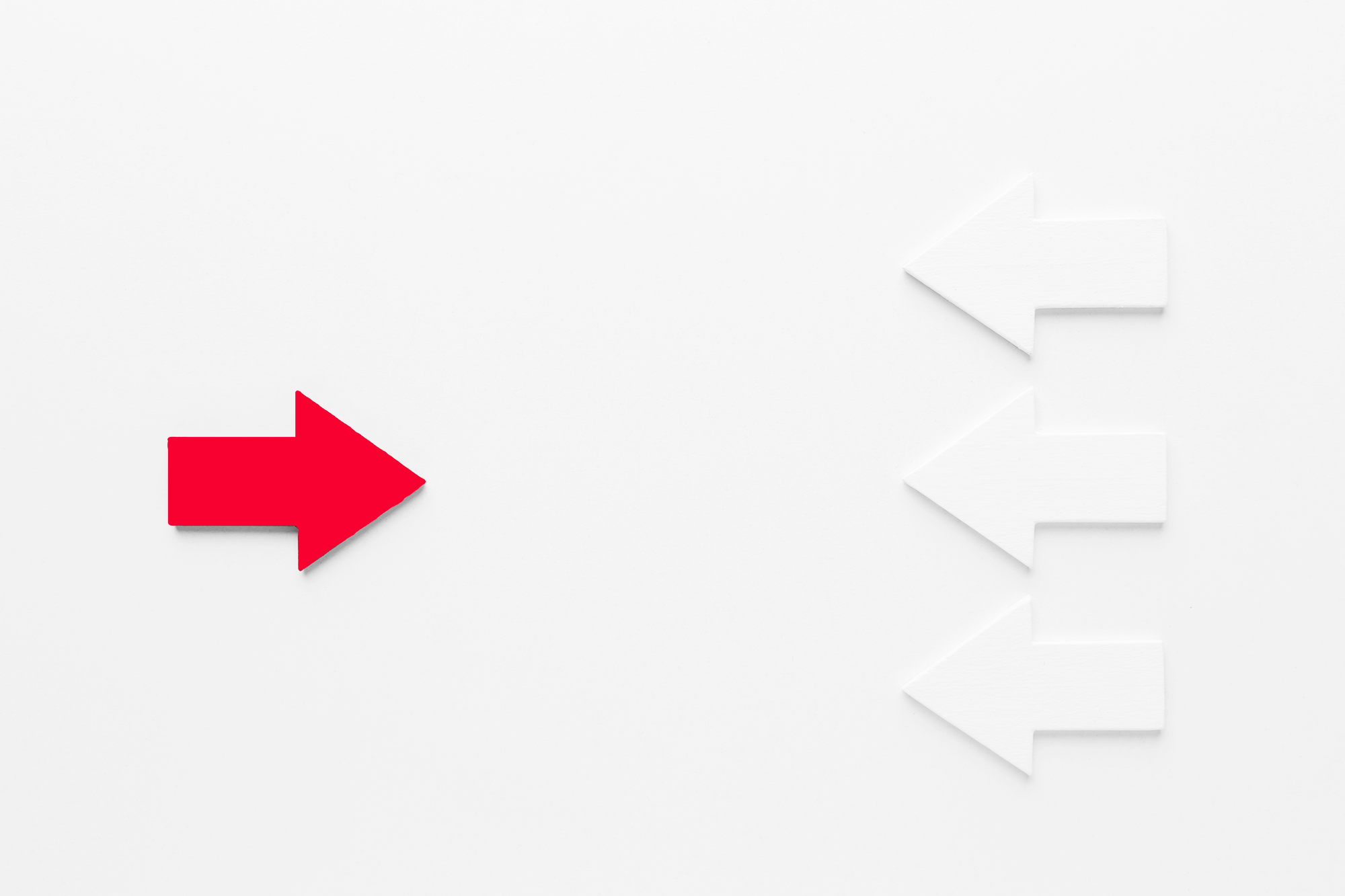